In this post, AI expert Jing Hu provides the lowdown on DeepSeek and the state of AI. Check out the article and some more of her great deep dives on her Substack here.
Let’s start with a question that might sting a little. When did we all become so bad at thinking and focus solely on being panicked?
AI industry in panic after China’s DeepSeek overtakes ChatGPT and Meta— The Independent
or
DeepSeek tech wipeout erases more than $1 trillion in market cap as AI panic grips Wall Street— Business Insider
People sell/act first, think later (or never).
Algorithms amplify fear, headlines scream about China’s AI leap, and everyone’s left scrambling to separate reality from runaway hype.
Here’s how one shockwave enhances the next:
It is much easier to hit “sell” or repost how DeepSeek makes Sam Altman panic than to ask, Wait—what exactly are we panicked about?
This isn’t about dismissing concerns.
I simply refuse to drown in the noise.
This article won’t spoon-feed you with my conclusion. Instead, I will walk you through my thought process while interrogating you with guided questions in clear categorization. You get to pick what is most critical to you.
Very simple, three parts:
Finally, a Survival Toolkit that sums up the red flags to spot via a mindmap template for you to download and expand your own thoughts at your own pace.
It began in a Shanghai high-rise in 2021.
Liang Wenfeng, was a hedge fund manager who made a billion-dollar bet that would alter AI history.
As his team at High-Flyer Capital developed trading algorithms, Liang quietly ordered staff to acquire every available Nvidia A100 GPU in Asia. It is estimated he hoarded 10,000 chips through shell companies for faster stock predictions. At least, that was the plan in 2021.
Then, in 2022, the Biden administration restricted the export of advanced computing chips.
The Pivot
2023, Chinese regulators cracking down on quant funds. Liang stunned investors by redirecting 90% of their assets into an AI startup. Skeptics walked out; true believers stayed to build DeepSeek.
Trial by Fire
Rumor says the early prototypes leaked in 2024 through a junior engineer's GitHub account. But of course, this is a rumor. What we do know is that DeepSeek has polished open-source models that undercut rivals. Here’s their Github.
Breakthrough
The rise of DeepSeek has been nothing short of a disruptive underdog story. Think of it as “Temu of AI.”
In May 2024, DeepSeek shook up the industry by releasing DeepSeek V2 as an open-source model, sparking a full-scale price war… at least in China.
On December 26, 2024, they did it again. With DeepSeek V3, the prices are even lower, redefining industry expectations: $0.14 per million tokens.
Shock and Awe
On January 20, 2025, R1 was released. The news on everyone’s feed is that R1 architecture delivers OpenAI o1 level reasoning at 1/10th the compute cost. By Monday, January 27, 2025, triggers a $1T tech stock selloff (17% drop in Nvidia + 5.6% sector-wide decline) as investors question Nvidia's AI infrastructure dominance.
A basic understanding of why DeepSeek R1 is so special is the foundation for you and me to ask critical questions.
Three most important technical breakthroughs from DeepSeek’s R1 model. With no-brainer analogies, don’t worry, even if you aren’t a techie. Here’s the R1 Paper (only if you are interested, of course).
The "Teamwork" AI Design (Mixture of Experts— MoE)
Instead of a "jack-of-all-trades" AI like OpenAI o1, think of R1 as a team of specialized experts collaborating like a kitchen crew.
There’s a master chef (the teacher model) who then trains line cooks (small models) through knowledge distillation. So a powerful AI can run on your laptop while staying smart, like getting Michelin-star meals from your home kitchen at a low cost.
MoE focuses on modularity and specialization for efficient task handling; OpenAI o1 emphasizes deep reasoning within a single model framework optimized for complex problem-solving.
Self-Taught Reasoning (using only Reinforcement Learning)
DeepSeek’s AI learned to reason on its own by trial and error, without extra guidance. It was given problems (math, coding) and rewarded for correct answers and logical steps.
Think of teaching a kid to ride a bike or play puzzle by letting them figure it out and rewarding the kid if they’ve done well. In contrast, OpenAI o1 is more like providing a structured problem-solving method and refining and improving within that framework.
Predicting Multiple Steps Ahead (Multi-Token Prediction)
The old way is like a chess player who only thinks one move ahead. On the other hand, DeepSeek R1 is like a chess player thinking several moves ahead.
DeepSeek R1 predicts several words or steps at once. In comparison, OpenAI's o1 prioritizes deeper reasoning with step-by-step problem-solving.
Knowledge Condensing (Distillation)
Imagine you’re preparing for an exam. You have the option to go with a 1,000-page textbook or a 100-page study guide that captures the key points, summaries, and essential concepts.
While the study guide doesn’t include every detail from the textbook, it’s compact and efficient enough to help you perform well on the exam.
In AI terms, the textbook is the large, complex AI model (the "teacher"). The smaller, distilled model (the "student") is the study guide. The process of creating the study guide is knowledge distillation.
Key questions you should ask to separate DeepSeek reality from hype:
DeepSeek's claim of ultra-low training costs for their AI model has raised eyebrows in the tech community.
It is also evident in their report that the training cost has excluded costs associated with prior research, ablation experiments on architectures, algorithms, or data.
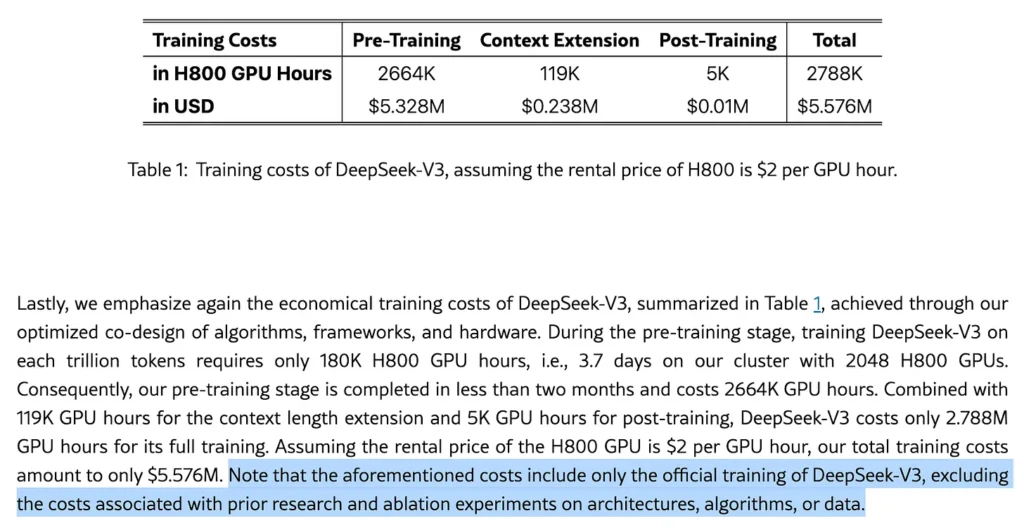
Still, the million as the unit of training cost starkly contrasts with the billions spent by US tech giants. If true (the H800 and the hours), it will disrupt the established business models. The precise methodologies underlying DeepSeek's breakthrough remain undisclosed.
There’s also a back-of-envelope calculation from an analyst that supports the low-cost claim.

Training cost comparison between DeepSeek V3 and Llama 3.1. DeepSeek, in theory, has no access (if not smuggled) to H100 GPU
Those who question the claim:
Some data scientists questioned whether DeepSeek had been developed with only 2,000 chips. They noted that the company might have operations with tens of thousands of Nvidia chips that could have been used in development.
Don't worry if you don't get any of these. The main takeaway is that, aside from the report by DeepSeek, no one yet can confirm their claim about training costs. It can be true… but we don't know until someone can replicate a similar model with an equally low cost.
Key questions for you to ask: What’s the catch of this low cost? Could there be a tradeoffs, e.g., What can’t this AI do well, and why?
Now, you’ve learned the foundation.
Shockwave #1: Major Tech Players.
DeepSeek R1 and more threaten the core premise of existing AI giants: dominance requires scale (more data, more compute, more $$).
If a lean Chinese startup can match OpenAI o1’s reasoning at 1/10th the cost, what does that mean for the "spend billions to win" playbook?
Shockwave #2: Chip Industry and Infracture.
Many news and influencers argue that Jevons Paradox will kick in. Simply put: Cheaper models → More startups (more AI usage) → More GPU buyers.
But what factors must exist for the Jevon paradox to be true? To say the least, it requires elastic AI demand, market needs, and other complementary resources to be available (e.g., engineers know how to build an efficient model as R1 does).
So questions you should ask:
Shockwave #3: Copyright Infringement and Data Privacy.
DeepSeek’s success raises tricky legal and ethical questions about who truly “owns” AI, the data, or the model used to train the next generation.
At the center of the debate is distillation (where a large model “teaches” a smaller model) and the copyrighted content used to train the earlier LLMs. Since the massive dataset is the fuel, where do privacy and intellectual property rights fit?
Questions to ask
Good read: OpenAI’s privacy policy. The first sentence in the policy:
When you visit, use, or interact with the Services, we receive the following information about your visit, use, or interactions…
DeepSeek’s policy, collects certain information when users set up an account).
It’s really about who you want to give your data to…The Chinese government or American commercial companies.
Shockwave #4: Startups and Smaller AI Firms
Yes, lower-cost training and inference could open doors for resource-strapped ventures. But does that also mean smaller players suddenly face a more crowded space, competing with DeepSeek itself and a throng of new entrants?
Shockwave #5: Enterprises and End Users
DeepSeek isn’t pushing AI to do anything dramatically “new” regarding raw capabilities. It’s distilling knowledge from existing large models at a fraction of the usual cost. Would This efficiency revolution trickle down to enterprises and end users?
Instead of a technical leap forward in intelligence by DeepSeek it is rather a cost revolution. However, that economic shift alone can significantly change how enterprises plan, deploy, and pay for AI. And how quickly people see tangible benefits every day.
Shockwave #6: Geopolitical and Regulatory
DeepSeek’s apparent success amid export controls spotlights a broader power struggle. Can bans on high-end chips (like Nvidia’s H100) actually curb AI development? Western nations face a choice: double down on regulations or adapt to a new competitive reality.
While others are still panicking about DeepSeek's efficiency breakthrough, the smart ones ask more profound questions about where this leads us.
What about the near-term future?
The Real AGI Question R1-Zero's self-training capabilities raise fascinating possibilities. But instead of asking, "Is this AGI?" ask:
What happens when AI can self-learn and self-improve at a fraction of today's cost?
The real opportunity in DeepSeek's breakthrough isn't just about cheaper AI. It's about who can ask the right questions and act accordingly.
Here’s the mindmap for you: Survival Toolkit.
Learn more about AI in product on Mind the Product by visiting our AI Knowledge Hub.