In the dynamic world of technology, the swift advancement of large language models has captured the attention of companies eager to integrate these innovations into their product strategies. The remarkable rise of ChatGPT has become a focal point, prompting teams to explore integrating chat experiences into their products. Recently, we’ve witnessed an upsurge in chatbots powered by these advanced AI models, carving success stories across various sectors. Notably, Whoop’s AI Coach in fitness and Replika’s AI chatbot in mental health have set benchmarks in their respective domains.
However, this journey hasn’t been without its challenges. Some companies have faltered in their rush to launch AI experiences, possibly due to inadequate user research. A case in point is Snap’s introduction of AI tools to their social media user base, who were left perplexed about their utility. This hasty integration, which needed an opt-out option for non-premium users, faced backlash and raised privacy concerns, particularly for younger demographics.
In 2024, Generative AI features will likely be prominently listed on the roadmaps of numerous companies. The challenge lies in deploying it to enhance product value, a topic becoming a staple in boardroom discussions and product planning sessions. However, achieving this is a nuanced endeavor. It demands a deep understanding of this novel technology and an appreciation of how these robust systems can transform user interaction with the products we interact with daily.
The emergence of ChatGPT marked a pivotal moment in the realm of Generative AI, an occurrence even OpenAI hadn’t fully anticipated. Engrossed in the incremental advancements of their models, they scarcely grasped the profound impact an accessible interface to the GPT model would unleash upon the masses. Although the leap from GPT 3 to 3.5 was more evolutionary than revolutionary, the real game-changer gave laypeople a gateway to harness this power. Once again, it was the simplicity and intuitiveness of the user interface that revolutionized the user experience.
Chatbots are emerging as a quick manifestation of Generative AI as organizations begin their foray into this new technology. This inclination towards chatbots is underpinned by three compelling reasons: the success narrative of ChatGPT, the existing infrastructure of chatbots in many companies, and their alignment with familiar communication formats. ChatGPT’s success story is well-documented and has become a persuasive argument for leveraging chat as an optimal means to access these sophisticated language models. This concept is evolving with the recognition that chat interactions need not be confined to text, as evidenced by the advent of multi-modal models.
Chatbot experiences have been gaining traction in numerous organizations over the years. While some, like Wells Fargo with their Fargo bot, have seen significant efficiency gains in customer service and other domains, many still leave much to be desired. Their limited functional scope is an expected shortfall, which offers marginal value over traditional interaction methods.
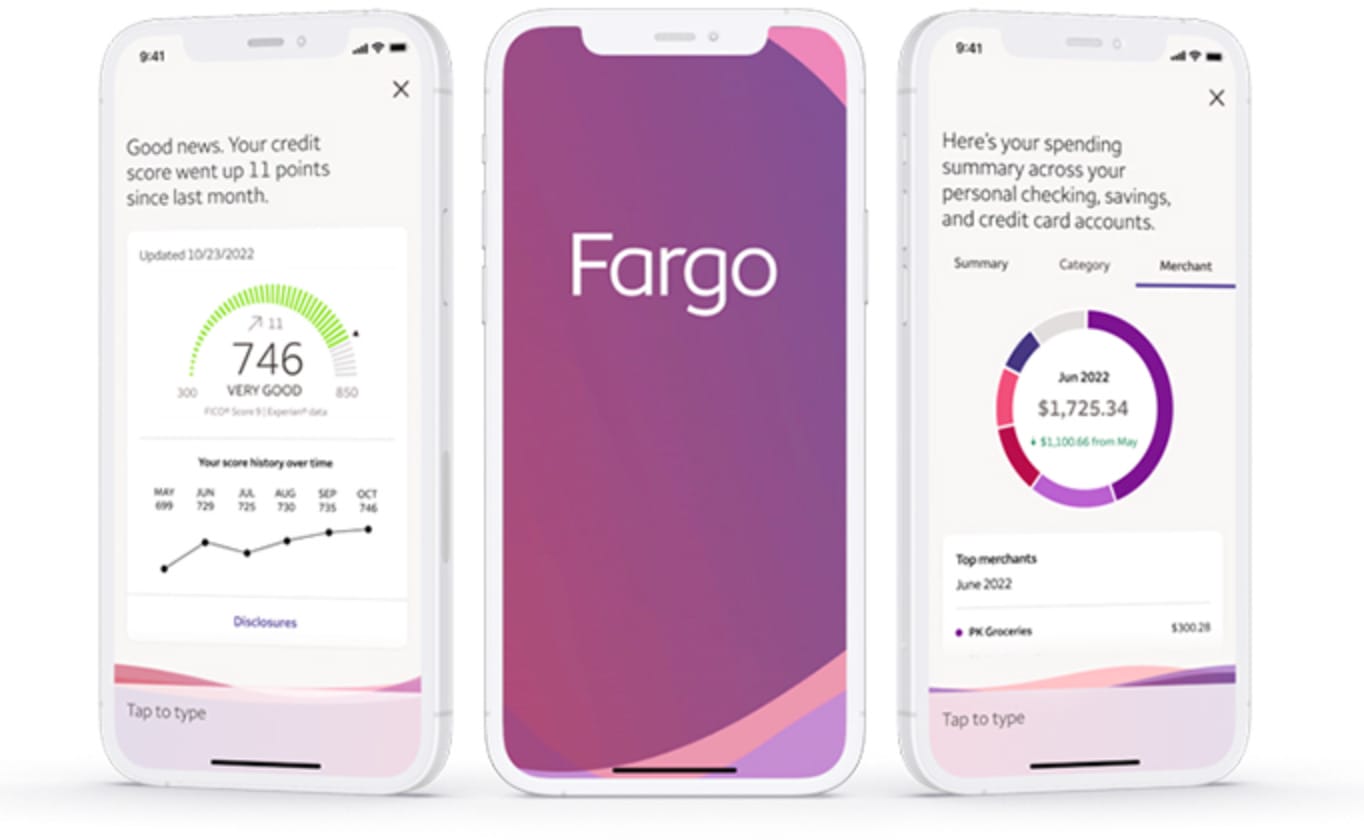
The Fargo bot by Wells Fargo has gained traction as an aid to quickly address banking needs
Their natural, conversational interface is the third driving force behind the growing preference for chatbots. Our societal interactions are deeply rooted in conversation, and chat systems, when adeptly designed, can seamlessly blend into the daily routines of users and workers. Consider the onboarding process of platforms like Slack or Figma versus ChatGPT. While the former requires users to adapt to new mental models for communication or creation, chat interfaces offer a more intuitive, conversational interaction. This doesn’t negate the need for onboarding but suggests a more straightforward, user-friendly approach.
Given these factors — the success story of ChatGPT, established chat infrastructures, and the innate familiarity with conversational formats — companies are likely to seriously contemplate chatbot development as a strategic component of their Generative AI roadmap. However, the true success of these chatbots depends heavily on the nature of their creation and contextual implementation. Design excellence is crucial; mastering it could be the key to fulfilling organizational objectives.
While the capabilities of new Large Language Models (LLMs) are groundbreaking, the concept of conversational systems is not new. There’s a wealth of scientific literature on developing conversational agents and ensuring they achieve their goals. This recognition extends to a broader spectrum of research on effective communication, initially intended for human interactions but primarily applicable to human-bot conversations as we are still in the early days of this technology; when in doubt, you can always consult these bodies of knowledge.
Drawing from my extensive experience as the lead user researcher for Generative AI products, alongside a thorough review of relevant literature, I’ve identified emerging trends that maximize the value derived from these systems. Building on the foundational work of others, I will share nine best practices for enhancing the user experience of chatbots. These practices are organized into three distinct categories:
Chatbot conversations: Focusing on the nuances of user interactions with the chatbot.
Building chatbot UIs: Centered on the design and functionality of the chatbot’s user interface.
Chatbot Deployment Strategy: Addressing the strategic integration of chatbots into existing products for a seamless user experience.
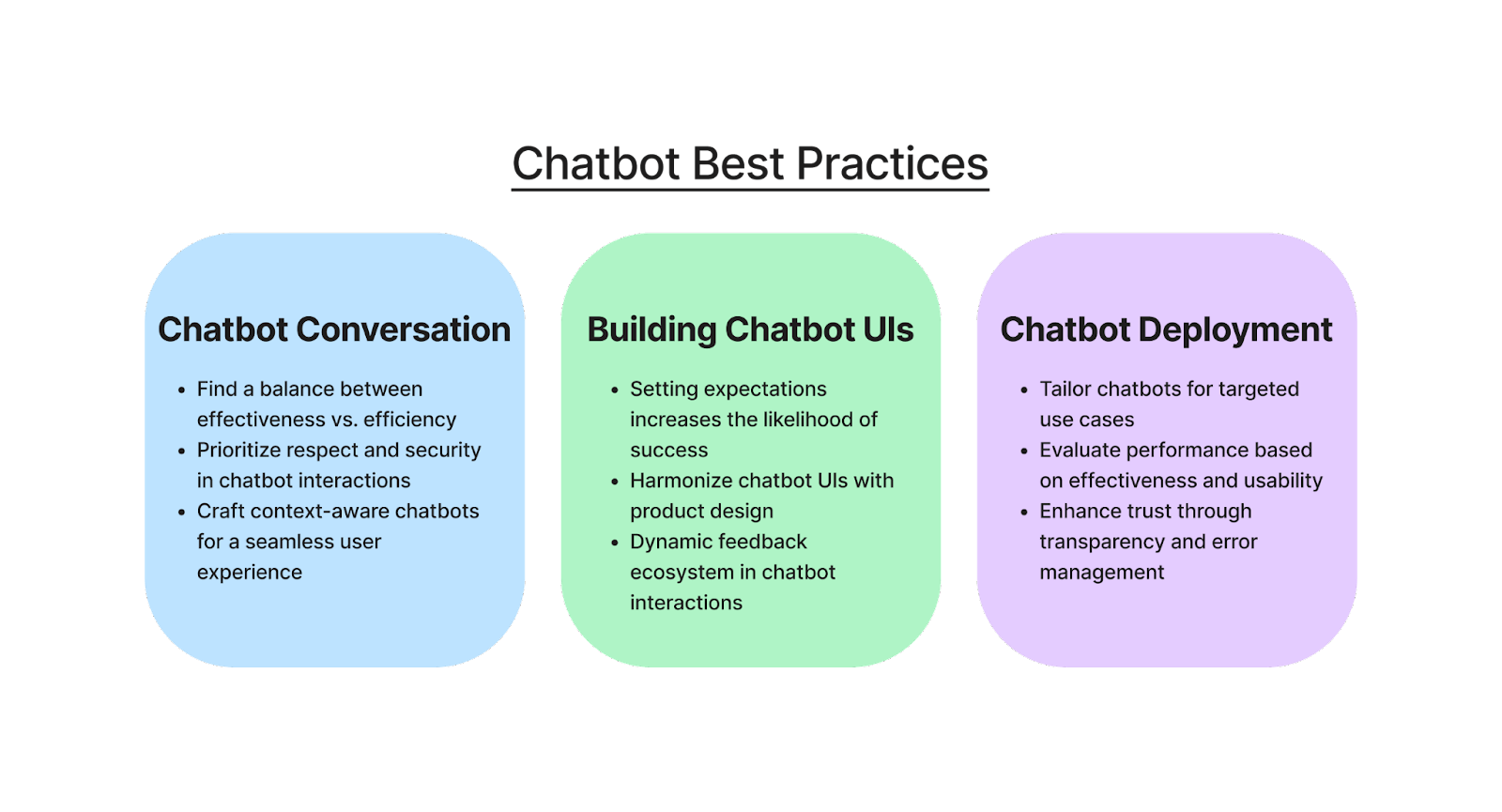
Three chatbot best practices categories with three recommendations in each.
Amidst the vast ocean of academic and practical research, several pivotal lessons have emerged concerning the essence of chatbot conversations. Teams venturing into chatbot development must swiftly grasp these insights to craft compelling dialogues. Training a chatbot involves defining its tone, programming its responses, and steering clear of sensitive topics. Striking the right chord in these areas is central to developing an engaging and effective chatbot conversational experience. Here are three best practices that serve as guiding lights in this endeavor:
In chatbot development, two key metrics stand out in measuring success: the effectiveness in accomplishing a given task and the efficiency of the interaction journey. This dual focus is particularly crucial in chatbot design, as it’s essential to develop a functional solution and ensure it conveys the right amount of information optimally timed.
Effectiveness consists of crafting solutions that deliver results. The essence of effectiveness in a chatbot is its ability to fulfill its intended function. Consider a chatbot designed to help users understand and alleviate stress. Assessing whether users conclude their interaction with a clear, actionable step towards stress relief is imperative. A combination of feedback surveys and transcript analysis is invaluable to gauge this. They provide insights into both the objective effectiveness of the chatbot and the users’ subjective experiences. The goal is to build a chatbot that users can confidently rely on for solutions.
On the other hand, efficiency is about creating an engaging user experience that accomplishes objectives in a timely fashion. The interaction with the chatbot should feel natural and fluid, without unnecessary delays or digressions. For instance, a chatbot guiding users in 401k allocation should provide sufficient information for informed decision-making yet avoid overly detailed digressions that could lead to user disengagement. The key is to strike a balance between thoroughness and brevity.
Navigating the trade-off between effectiveness and efficiency is a delicate process. These two aspects often pull in opposite directions, making user research indispensable for teams. It is critical to understand user interactions with the chatbot and their perceptions of these interactions. New systems are spawning up that help with the creation of chatbot analytics with ItsAlive being a great example of the initial metrics associated with measuring efficiency such as average session duration. These data enable product leaders to make informed decisions when adjusting prompts for greater clarity and effectiveness or streamline them for increased efficiency. This balance is the cornerstone of a successful chatbot experience that meets and exceeds user expectations.
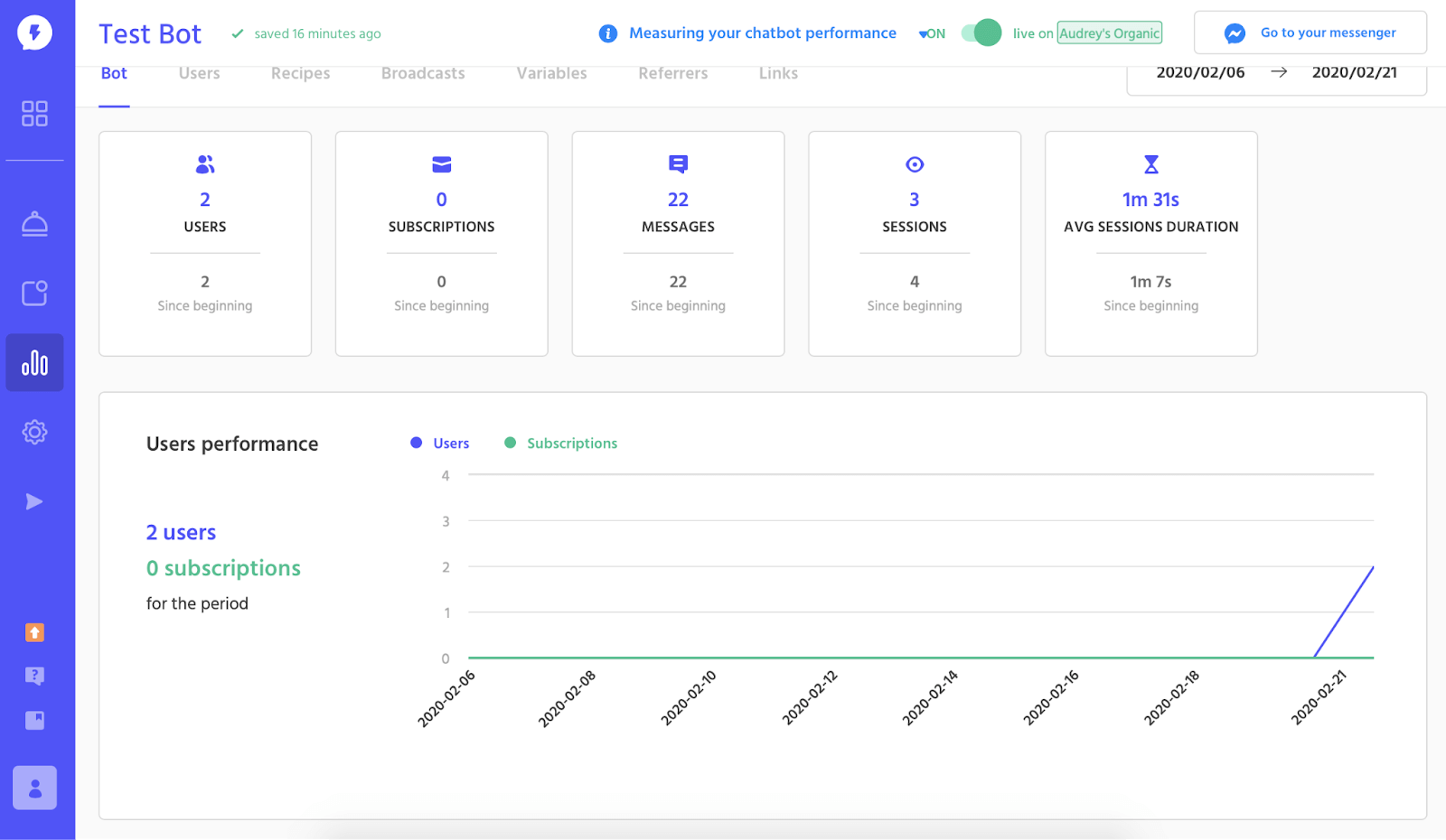
ItsAlive offers a dashboard that provides basic analytics about chatbot interactions.
In the artificial intelligence landscape, chatbots’ integrity and impact are heavily influenced by the data on which they are trained. Faulty or biased data can perpetuate existing societal biases, leading to detrimental effects, mainly when these models are applied in sensitive areas like loan approvals, where biases against marginalized groups have historically been a concern. The risk escalates when considering chatbots engaged in live, dynamic conversations, where users might share profoundly personal or sensitive information.
To address these challenges, companies developing chatbots must emphasize reducing bias and safeguarding data privacy throughout the development process. Although foundational models like GPT have undergone extensive risk assessment, it’s imperative for specific chatbot applications to undergo further scrutiny. This ensures that they do not inadvertently discriminate against any protected customer groups, particularly in the unique contexts in which they are deployed. Furthermore, it’s not just about securely storing conversation transcripts; the same level of security and privacy must extend to any data extracted or used from these interactions.
Building upon these technical considerations, teams must also design chatbots to foster a respectful and secure user environment. This approach involves diligently mitigating social and cognitive biases and guaranteeing that all interactions are secure and private. These conversations must be programmed to uphold the user’s autonomy and dignity. This dual focus on technical accuracy and ethical interaction is vital in creating chatbot experiences that are effective, deeply respectful, and secure, aligning with the highest standards of user respect, privacy, and security.
In human conversations, context is everything. It shapes our interactions and expectations, a principle that holds even more weight in critical or sensitive discussions. For chatbots to be effective, they must exhibit a similar level of contextual awareness, adapting to a user’s profile and the specifics of the ongoing interaction. For instance, a savings chatbot should be mindful of a user’s financial history, like past struggles with debt, to avoid making them feel disregarded or upset. Similarly, a relationship advice bot must be sensitive to recent significant events in a user’s life, such as a breakup, to maintain an appropriate tone.
Large language models, brimming with insights into the human experience, often do a commendable job of approximating human emotions and reactions. Harnessing this capability requires crafting prompts that allow for dynamic, context-sensitive conversations, considering the user’s background and immediate experiences. The ideal scenario involves integrating past interactions and current activities within the broader product ecosystem to construct a comprehensive user profile.
In aligning with these principles, AI-driven chatbot interactions should fluidly adapt to various factors: the user’s identity, their history of interactions, and the broader contextual environment. This requires enabling users to provide direct feedback, allowing the AI to make real-time adjustments. The chatbot’s personality should evolve in harmony with the user, learning from and adapting to their behavior in an engaging but not overbearing manner. Each message should be uniquely tailored, displaying information relevant to the individual’s context.
Furthermore, the tone and even the interface of the chatbot should be flexible, adjusting in response to user feedback. Crucially, the bot should be able to recall recent interactions and provide easy access to the conversation history. This approach ensures a more personalized, context-aware, and fluid chatbot experience, mirroring the nuances and adaptability of human-to-human interactions.
While the development of Chatbot User Interfaces (UIs) can essentially draw upon the extensive reservoir of traditional user experience research and knowledge, the unique nature of interacting with advanced, sophisticated bots introduces fresh challenges and considerations. To enhance the chances of a successful deployment, there are specific best practices tailored for Chatbot UIs that I recommend:
Introducing new users to a platform marks a critical phase where initial impressions and mental models are formed. This stage often dictates the user’s perception and interaction with the platform for a considerable duration. Introducing a chatbot feature follows this rule but with added layers of complexity, particularly in expectation management. With many users already familiar with the broad accessibility of platforms like ChatGPT and BARD, any specialized chatbot must communicate its intended purpose. This clarity plays a vital role in paving the way for user success.
Setting expectations is not just about clarifying the chatbot’s function; it’s an opportunity to shape the user’s perception of the bot’s performance. Research from MIT and Arizona State University demonstrates the power of framing in influencing users’ trust, empathy, and perceived effectiveness of chatbots. Therefore, when constructing the onboarding experience for a chatbot, it’s crucial to craft the narrative carefully. Begin with the desired outcomes for the user, then strategically weave these into the language and context used during the onboarding process.
In line with these insights, it is essential to provide users with a thorough introduction to the AI chat format, delineating its capabilities and limitations. A vital aspect of this is to acknowledge that generative AI, despite its sophistication, is not infallible and can make errors. Assisting users in crafting effective prompts is part of this educational process. The onboarding phase should familiarize users with the conversational interface and set realistic expectations regarding the chatbot’s functionality.
For instances where the chatbot is tasked with novel or complex functions, step-by-step explanations are invaluable. This guided approach helps users navigate new tasks with ease and confidence. The introduction of the chatbot should be structured akin to a conventional onboarding process, placing significant emphasis on understanding the chatbot’s limitations and capabilities. This structured approach to onboarding is pivotal in fostering an informative and empowering user experience, setting the stage for a productive and satisfying interaction with the chatbot.
Incorporating chatbots into the broader product experience demands a nuanced approach to design. The key lies in achieving a delicate balance: the chatbot feature should seamlessly blend with the product's overarching design ethos while simultaneously distinguishing itself as a unique, chat-based interaction. This dual objective ensures users transition smoothly into the chatbot experience, maintaining a sense of continuity yet being mindful of engaging with a distinct, chat-centric feature.
Achieving this balance is crucial. It allows users to retain the context of their ongoing activity while preparing them for the unique dynamics of interacting with a chatbot. Consider, for instance, a marketing software where standard features involve selecting templates, customization, and campaign execution. Introducing a chatbot into this environment can expedite processes and introduce new interaction paradigms. Users should perceive that they are still within the same workflow but also be alert to the nuances of engaging with a chatbot — like being vigilant for potential misunderstandings and meticulously reviewing AI-generated content for quality assurance. Incorporating a disclaimer about the generative AI nature of the chatbot can also serve as a best practice, setting clear expectations about its capabilities and limitations.
In line with these considerations, chatbot integration should be executed to complement the overall product design, ensuring contextual relevance and ease of use. This entails developing UIs that are flexible, adaptable, and uncluttered, minimizing reliance on extensive text or instructions. The chatbot’s activation should be contextually timed, triggered by specific user actions or at opportune moments, and users should find it straightforward to engage or disengage with the AI systems. By thoughtfully integrating these elements, the chatbot becomes an organic product extension, enhancing the user experience through its intelligent, context-aware presence. One example is Priceline’s Penny bot which fits the same font, color scheme, and style of the rest of the website.
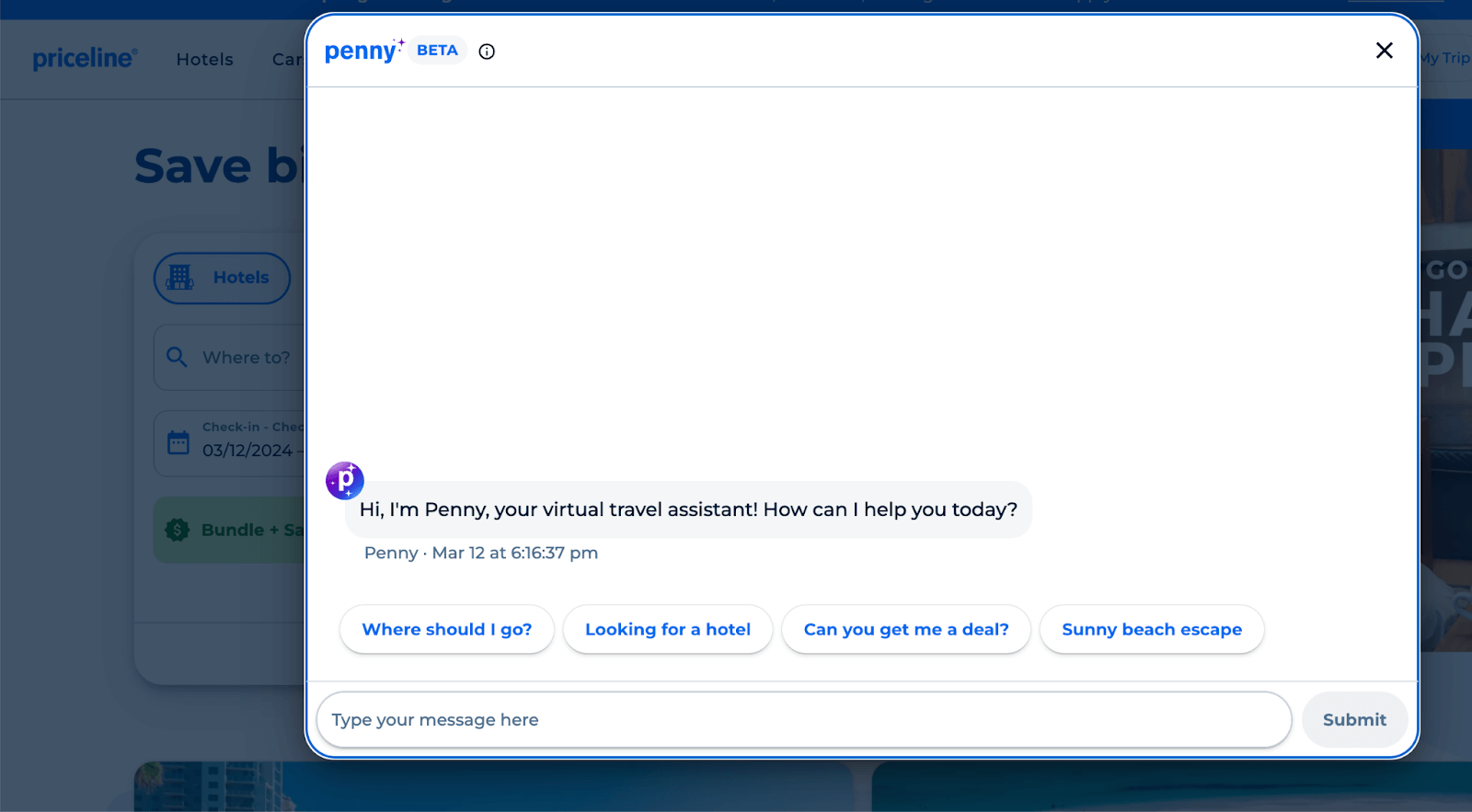
Priceline’s Penny bot fits the same font, color scheme, and style of Priceline’s Design System.
In the continuous evolution of products, user feedback stands as a cornerstone, especially vital in refining chatbot experiences. Adhering to the best practices of soliciting user input, particular emphasis should be placed on gathering insights about the effectiveness of the chatbot conversations. Strategically placing feedback buttons within the user’s visual field when interacting with the chatbot is practical. However, the importance of this practice extends beyond mere collection — actively monitoring and responding to this feedback is crucial, given the ever-evolving nature of the underlying models of many chatbots and the varying outcomes they generate.
Parallel to extracting user feedback, an often less familiar yet equally important aspect is providing updates back to the users. Since the prompts and algorithms driving these chatbots can undergo frequent updates, keeping users informed about significant changes is imperative. This proactive communication can avert confusion or dissatisfaction arising from unexpected shifts in the chatbot’s behavior or outputs. Notifications of such updates can be seamlessly integrated into the chat flow for minor changes, while more substantial updates warrant more direct communication methods, like pop-ups or dedicated notifications.
This approach establishes a bidirectional feedback loop, where users contribute their insights and stay informed about the chatbot’s evolution. Transparency in communicating AI system updates, including their rationale, is critical to maintaining user trust and engagement. Users should have easy and intuitive channels to provide feedback, mainly when the AI system falls short of expectations. Moreover, if a user action leads them away from the chat interface, a gentle nudge to review and provide feedback on the AI’s performance can be beneficial. This holistic feedback ecosystem, characterized by mutual exchange and transparency, is instrumental in enhancing the user experience and continuously improving chatbot functionalities.
Deploying a chatbot might appear as a straightforward task where a learn-as-you-go approach suffices. However, considering the inherent unpredictability of these AI systems and the criticality of making a positive first impression, a more deliberate and strategic approach is advisable. This helps mitigate potential risks and sets the stage for a successful launch. Here are three recommended best practices for crafting an effective chatbot deployment strategy:
The widespread success of general-purpose chatbots, notably ChatGPT, as seen in early 2024, shouldn’t mislead organizations into overlooking the significance of specialized, purpose-built chatbot solutions. These tailored chatbots are designed to address specific user needs or provide targeted information, which often aligns more closely with the strategic objectives of many organizations. Ideally, an organization should align chatbot development with its list of user requirements, viewing chatbots as a versatile tool for addressing these needs.
When designing a chatbot, the team must deeply understand one or two specific use cases the chatbot will address. This involves setting clear objectives for the chat experience and defining metrics to gauge the chatbot’s effectiveness in meeting these goals. Taking a focused approach enables the development team to craft precise prompts that effectively fulfill specific needs rather than attempting to cover too broad a spectrum with minimal impact. Moreover, having a well-defined purpose for the chatbot facilitates transparent communication with users about its capabilities and limitations, as highlighted in the onboarding best practices. This transparency is essential for managing user expectations and enhancing the overall experience.
An example of a finely tuned specific chatbot is BetterUp, who has created an AI experience that can intervene with a user for a variety of situations. One of these is a role playing scenario bot intended to help the user prepare for a difficult conversation that they will be facing in their life. Given the tailored situation this bot is being deployed within, the team can create specific effectiveness measurements, such as how prepared a user feels as determined by the chat transcript and how confident they are going into their conversation.
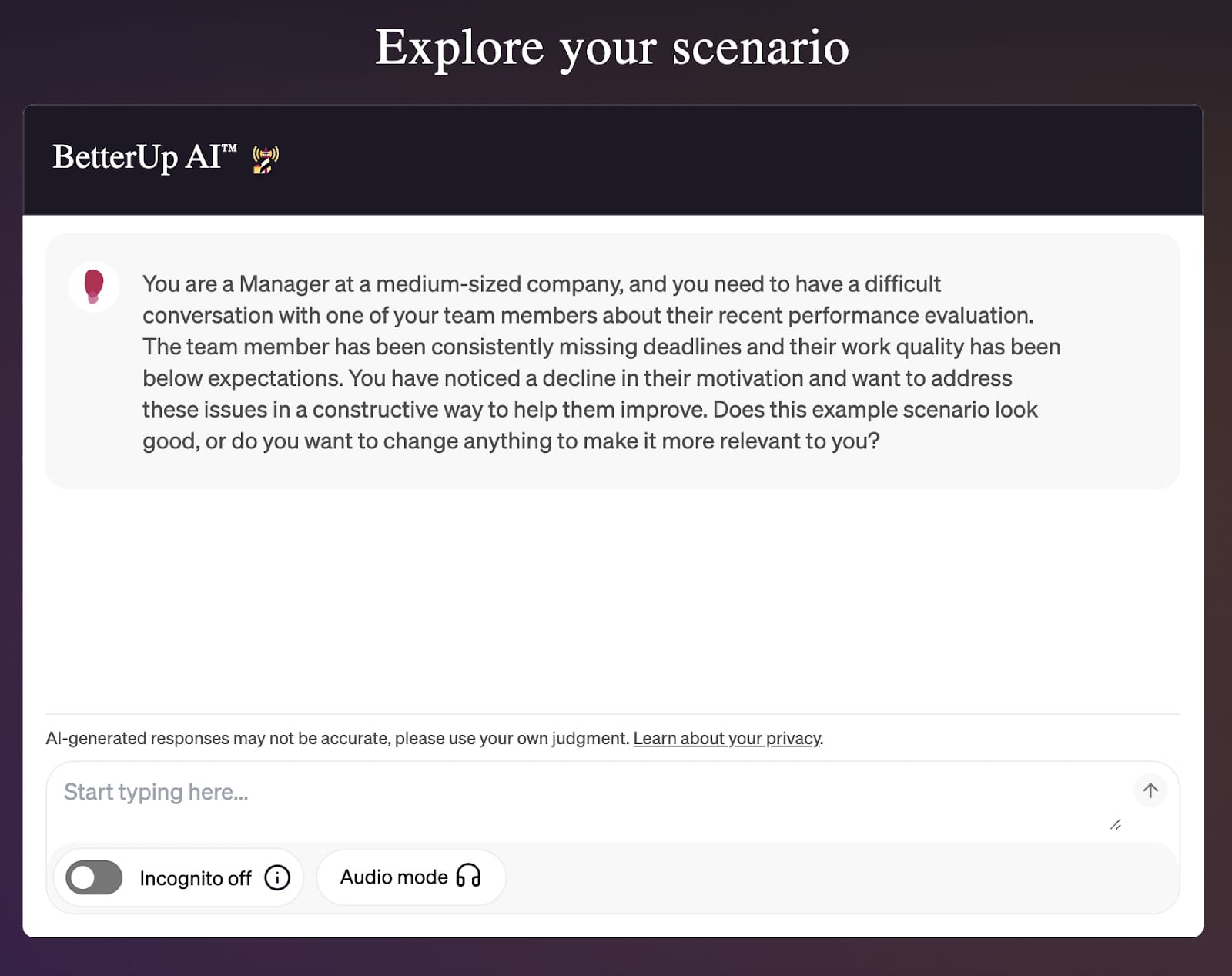
BetterUp has built a Chatbot tailored to assisting the user prepare for a difficult conversation.
Even general-purpose chatbots should be developed with a clear intention, focusing on facilitating natural interactions that directly resolve user issues. The chatbot should concentrate on specific use cases to achieve the desired outcomes effectively. Establishing and communicating clear boundaries about the AI system’s capabilities is also vital to ensure that users have a realistic understanding of what the chatbot can and cannot do. Taking this approach enhances user satisfaction and contributes to the organization's strategic and efficient deployment of chatbot technology.
For any feature launch, including chatbots, establishing and monitoring key performance indicators is essential for measuring success. Chatbots, in particular, offer the advantage of being highly adaptable with minimal effort. Unlike traditional features requiring extensive coding and interface adjustments, chatbots can undergo significant changes simply by updating the underlying prompts. Therefore, it’s critical to have metrics that assess both the effectiveness in addressing the primary use case and the overall usability of the chatbot. These measurements guide teams in making informed decisions about necessary modifications or even the potential discontinuation of the chatbot.
To gauge the effectiveness of a chatbot, two main approaches can be employed: direct communication with users and analysis of conversation transcripts. Such an analysis can be conducted by human reviewers or through AI-driven metrics, such as those provided by GPT. For instance, a chatbot designed to assist in creating dating profiles can be evaluated based on its success in generating more matches, complemented by user feedback on satisfaction with the profiles it produces. A more intricate analysis might involve using GPT to analyze conversations and assess changes in user confidence or overall sentiment throughout the interaction. Usability assessment of the chatbot involves examining how users respond to the bot’s phrasing and questioning, akin to standard usability evaluations for other features. Taking this approach involves looking into user reactions and adaptations to the conversational style and flow dictated by the chatbot.
In essence, the success of a chatbot hinges on its problem-solving effectiveness and the enjoyment users derive from their interactions. Therefore, the primary metrics for evaluation should focus on the functional effectiveness of the bot and the degree of user satisfaction it elicits. Additional crucial indicators include the relevance and understandability of the bot’s responses, which are pivotal in determining its overall efficacy. By closely monitoring these aspects, teams can continually refine the chatbot, ensuring it meets and exceeds user expectations and requirements.
Transparency in decision-making and effective handling of mistakes are critical components of chatbots that significantly influence user trust and acceptance. While the underlying models of these bots often function as black boxes, teams can still foster transparency by communicating the rationale behind certain decisions and updates made to the bot. When a conversation leads to a specific outcome, users should be informed, as much as possible, about the factors that influenced the bot’s decision. Enabling users to provide input or feedback on these decisions builds trust and enhances their acceptance and satisfaction with the outcomes.
Mistake handling is an inevitable and crucial aspect of managing chatbots. Teams should have robust systems to identify and correct errors the bot makes. Beyond technical rectifications, it’s essential to communicate with affected users, providing explanations for any issues or inappropriate responses they may have encountered. This level of transparency in acknowledging and addressing errors is vital to maintaining user trust. Chatbot.com has distilled recommendations coming from a leader in the design field NN/g, into five recommendations as shown in the following image.
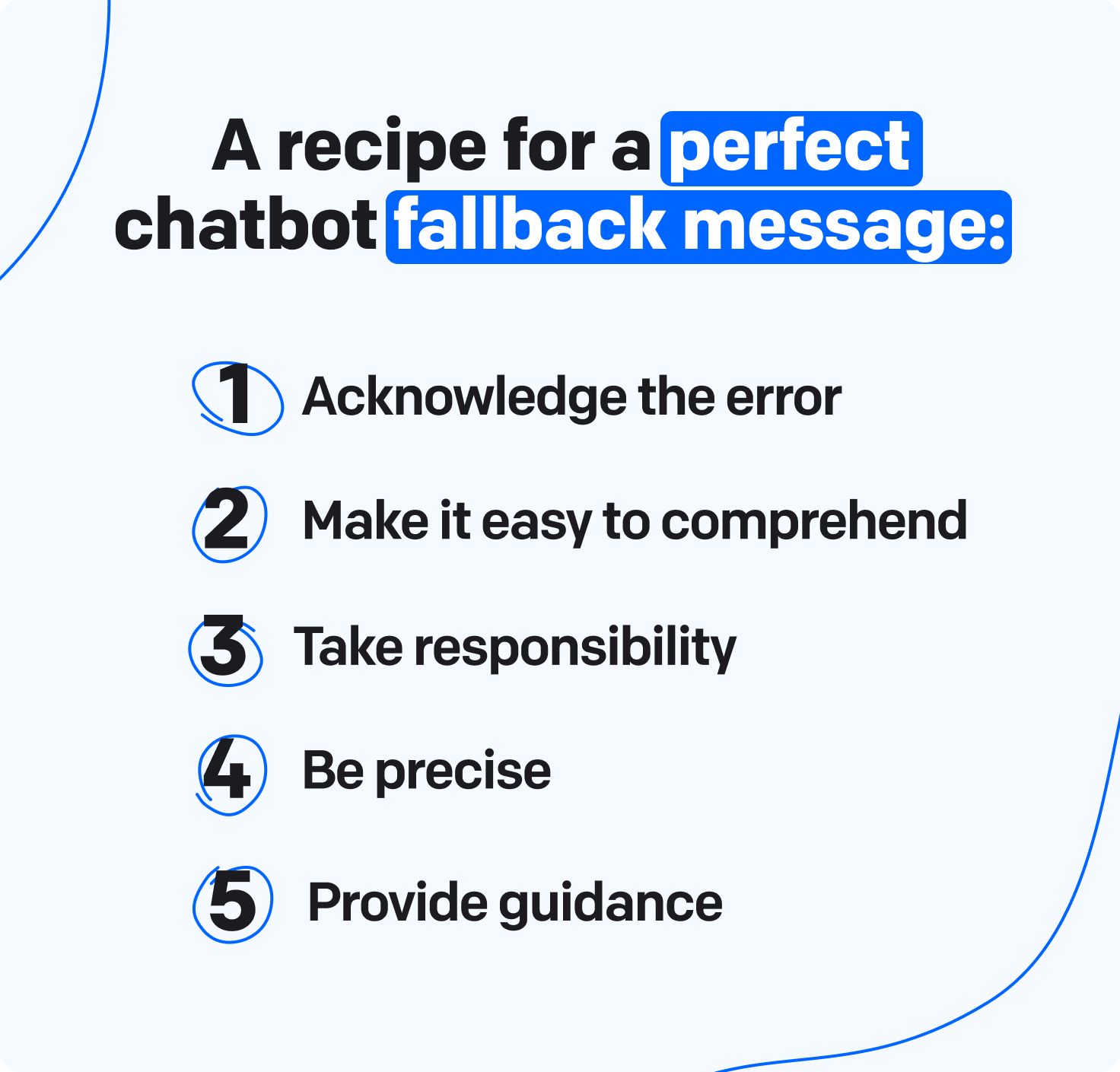
Photo Credit: www.chatbot.com. The five rules to build a strong chatbot error message.
Additionally, empowering users to reset conversations or backtrack on specific inputs can significantly enhance their experience. This feature allows users to correct mistakes or misunderstandings, fostering a sense of control and ownership over the interaction. The more autonomy users have in guiding and correcting the course of the conversation, the more likely they are to integrate the chatbot into their regular workflow.
In summary, users require a clear understanding and insights into the decisions made by AI systems. Correcting AI-driven outcomes should be straightforward and intuitive, enabling the user and the system to rectify misunderstandings efficiently. By implementing these practices, chatbots can achieve higher levels of user trust, satisfaction, and effectiveness, ultimately leading to a more harmonious integration of this technology into users’ daily lives and routines.
Conversational interfaces represent a natural way of interaction, yet typing responses is only sometimes the most efficient or intuitive method of communication. There’s an emerging trend towards voice-based features, aligning more closely with the concept of a personal assistant interface. The growing enthusiasm for multi-modal models stems from an understanding that human interactions are not limited to text but encompass visual and auditory elements. Future chat interfaces are likely to embrace this reality, supporting a variety of interaction styles and preferences.
While chatbots currently stand as a prominent application of generative AI, it’s essential to recognize that they represent just one of many potential interfaces. A notable analysis by Emergence Capital explores various manifestations of generative AI beyond chatbots. They categorize chatbots into general and specialized types, as discussed earlier in this article. Beyond chatbots, they identify categories like Co-Pilots and AI-enhanced features. Co-Pilots, as exemplified by Microsoft Co-Pilot, leverage AI to augment tasks like document creation. Although adopted more gradually, AI-enhanced features are effectively demonstrated in platforms like Notion AI, which integrates AI to enrich various functionalities.
Chatbots, while potentially a mainstay in communication, might also be transient, serving as a bridge to more advanced modalities. Regardless of their future trajectory, chatbots are significant in many companies’ 2024 roadmaps. The key to their successful implementation lies in ensuring they are not only usable but also practical. This involves designing chatbots that not only fill current gaps but also pave the way for more comprehensive AI-driven features. Whether chatbots remain a staple or evolve into something else, their development today is a step towards enriching user experiences with AI, heralding a future where technology seamlessly integrates with natural human interaction.
Explore more great product management content by exploring our Content A-Z